Big biological datasets map life’s networks
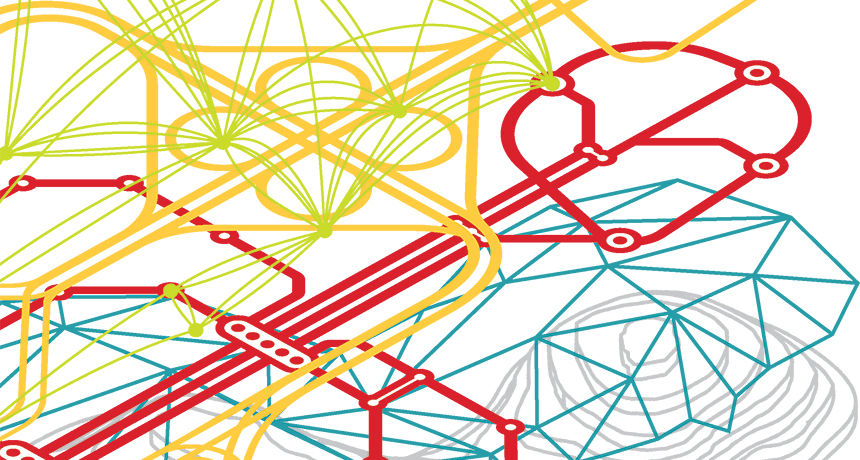
Michael Snyder’s genes were telling him that he might be at increased risk for type 2 diabetes. The Stanford University geneticist wasn’t worried: He felt healthy and didn’t have a family history of the disease. But as he monitored other aspects of his own biological data over months and years, he saw that diabetes was indeed emerging, even though he showed no symptoms.
Snyder’s story illustrates the power of looking beyond the genome, the complete catalog of an organism’s genetic information. His tale turns the genome’s one-dimensional view into a multidimensional one. In many ways, a genome is like a paper map of the world. That map shows where the cities are. But it doesn’t say anything about which nations trade with each other, which towns have fierce football rivalries or which states will swing for a particular political candidate.
Open one of today’s digital maps, though, and numerous superimposed data sources give a whole lot of detailed, real-time information. With a few taps, Google Maps can show how to get across Boston at rush hour, offer alternate routes around traffic snarls and tell you where to pick up a pizza on the way.
Now, scientists like Snyder are developing these same sorts of tools for biology, with far-reaching consequences. To figure out what’s really happening within an organism — or within a particular organ or cell — researchers are linking the genome with large-scale data about the output of those genes at specific times, in specific places, in response to specific environmental pressures.
While the genome remains mostly stable over time, other “omes” change based on what genes are turned on and off at particular moments in particular places in the body. The proteome (all an organism’s proteins) and the metabolome (all the metabolites, or small molecules that are the outputs of biological processes) are two of several powerful datasets that become more informative when used together in a multi-omic approach. They show how that genomic instruction manual is actually being applied.
“The genome tells you what can happen,” says Oliver Fiehn, a biochemist at the University of California, Davis. The proteome and the metabolome can show what’s actually going on.
And just as city planners use data about traffic patterns to figure out where to widen roads and how to time stoplights, biologists can use those entwined networks to predict at a molecular level how individual organisms will respond under specific conditions.
By linking these layers and others to expand from genomics to multi-omics, scientists might be able to meet the goals of personalized medicine: to figure out, for example, what treatment a particular cancer patient will best respond to, based on the network dynamics responsible for a tumor. Or predict whether an experimental vaccine will work before moving into expensive clinical tests. Or help crops grow better during a drought.
And while many of those applications are still in the future, researchers are laying the groundwork right now.
“Biology is being done in a way that’s never been done before,” says Nitin Baliga, director of the Institute for Systems Biology in Seattle.
Data dump
Scientists have long studied how genes influence traits. Researchers have figured out important connections between genes and the proteins they encode and have scoured the genome for associations between particular genetic mutations and diseases. But a gene-by-gene view of the body is like trying to diagnose a citywide traffic problem by looking at just one backed-up intersection.
“There are so many places that a system can go awry,” Baliga says. When dozens of genes are working together, it’s tricky to tease out which one is misfiring in a particular instance.
Baliga is among a growing group of scientists who want to study life through a systems lens, because sometimes that traffic jam at one intersection is being caused by an out-of-sight accident three blocks away.
Such an approach is particularly useful for unraveling the complexities of diseases like cancer and diabetes. These conditions involve a tangled web of genes, paired with lifestyle factors and environmental conditions — Is she a smoker? How much does she exercise? — that influence when those various genes are turned on and off.
Reconstructing the tangled routes by which genes interact to influence the body is a slightly more complicated feat than mapping the best path from Tulsa to Tuscaloosa. For one thing, it requires serious computer power to gather, store and analyze all that data. The 3 billion chemical coding units that string together to form a person’s inventory of DNA, if entered into an Excel spreadsheet line-by-line, would stretch about 7,900 miles. The human proteome contains more than 30,000 distinct proteins that have been identified so far. And researchers have cataloged more than 40,000 different human metabolites, such lactic acid, ethanol and glucose.
Working with such big datasets can be expensive, too. Compiling the first human genome took 10 years and cost almost $3 billion. Now, the costs of collecting and analyzing all these datasets have come down, so it’s finally feasible to use them in tandem to answer big biological questions.
The important players
Scientists would like to understand the interplay between the genome and the proteome. Add in the metabolome. To make things more complex, there’s the epigenome — the chemical modifications to DNA that help control which genes are turned on and off — and the transcriptome, the full range of RNAs that translate DNA’s blueprints so they can be used to make proteins. It’s no surprise that mapping such a comprehensive network for any organism is still a distant goal.
For now, scientists tend to focus their multi-omic studies on a particular disease or question. Baliga wants to learn how tuberculosis — which sickens nearly 10 million people per year and kills 1.5 million — evades drugs within the body. Many strains of the TB bacterium are resistant to existing treatments or can tolerate them long enough to establish a strong foothold.
To learn how Mycobacterium tuberculosis mounts a defense against a drug, Baliga is first looking within the bacterium, identifying the genes, proteins and other molecules that interact as the microbe infects a host.
He collects different types of omic data from M. tuberculosis alone and when it’s in the presence of an antibiotic. His team recently focused on the microbe’s response to bedaquiline, a drug used to treat multidrug-resistant TB. Baliga measured the microbe’s transcriptome in the presence of different doses of bedaquiline and at different times after introducing the drug.
From this giant data dump, computer models helped narrow the focus to a smaller collection of genes, proteins and other molecules that changed under certain conditions. Visualization programs turned these mathematical outputs into maps that scientists could analyze.
About 1,100 genes behaved differently in the presence of bedaquiline, Baliga’s team reported in August in Nature Microbiology. Measurements of the RNA indicated that most of those genes became less active, but a few shifted into overdrive. The researchers suspected those hyperactive genes might be behind the resistance — playing off each other to create a smaller network within the larger tuberculosis response network.
But statistical analysis alone wasn’t enough to confirm the hunch. Correlation isn’t cause, Fiehn points out. Scientists need to figure out which of those changes actually matter.
That is, if you’re scanning millions of data points looking for variation, you’re going to find certain abnormalities that are due to chance and are unrelated to the disease or
question at hand. But starting from that smaller dataset of outputs that change, scientists can then test which players are actually important in the network and which belong on the sidelines. In animal models or petri dishes, scientists disable one gene at a time to see how it affects the proposed network.
“Systems biology has been able to generate these amazing hypotheses about how genes interact,” Baliga says. Testing them has historically been more challenging. But now, gene-editing technologies such as CRISPR/Cas9 (SN: 9/3/16, p. 22) allow scientists to more easily test these networks in living systems.
Baliga and his team edited the genome of M. tuberculosis, disabling the regulatory machinery responsible for some of the overactive genes. Sure enough, the drug worked better on the modified bacteria, the researchers reported.
Networking solutions
Once a network has been mapped, scientists can use it to predict (and maybe even prevent) illness.
Baliga’s team identified a second drug that works with bedaquiline. The drug turns off some of the regulators for the overactive tuberculosis gene network that was fighting off the bedaquiline. Using the second drug with bedaquiline made tuberculosis bacteria more vulnerable, pointing to a potential strategy for dealing with persistent infections.
Baliga’s group is also mapping networks in patients with glioblastoma, a particularly deadly type of brain tumor. In the August Cell Systems, the scientists described work designed to figure out why some patients respond to certain drugs while others don’t. The aim is to personalize treatments, to choose a drug that targets the particular network glitch that gives rise to that patient’s tumor, Baliga says. The drug might ramp up production of a protein that’s currently in short supply, or turn off a gene that’s mistakenly on. That same drug might be completely useless for another patient whose tumor developed through a different network error.
“Being able to do that systematically across all cancers, using networks — that has not happened yet,” Baliga says. But scientists have devised drug treatments to address individual mutations. And expanding that to a greater range of cancers in the future is not farfetched, he says.
Other scientists are using multi-omic approaches for preventive medicine, for example, to be more effective and efficient in vaccine development. Despite years of trying, scientists still haven’t created an HIV vaccine that can protect people against the virus, says Alan Aderem, a biologist at the Center for Infectious Disease Research in Seattle. Bringing a vaccine from test tube to market is costly and time-consuming. With a better understanding of how the networks of the body’s immune system respond to the disease, researchers could be more selective in the vaccine candidates that they invest time and money in developing.
“The real value of systems biology is that it’s predictive,” Aderem says. “If you could predict upfront if a vaccine would work, you’d not only save a huge amount of energy, you’d also save a huge amount of lives.”
Plant power
Multi-omics has perhaps received the most attention in the context of human health — but that’s also the realm where it’s hardest to piece together the omic layers. Because simpler organisms can be manipulated genetically, it’s easier to move from networks on a computer screen to real solutions.
For instance, some scientists are using multi-omic analysis to engineer networks that let crop plants thrive with less water. To turn carbon dioxide into sugar via photosynthesis, plants need a lot of water. But some desert plants make do with less. Most plants take in carbon dioxide through small pores in their leaves. Opening these pores can let water evaporate out. In the desert, where water is in short supply, some plants use a different network of chemical reactions to make energy: crassulacean acid metabolism, or CAM.
Plants that use CAM open pores in their leaves only during the cooler nighttime, when water is less likely to evaporate out. They store the CO2 they take in until daytime, when they close the pores and convert the CO2 into food.
“Our goal is to move this metabolic trick into crop plants,” says John Cushman, a biochemist at the University of Nevada, Reno. “In order to do that, you have to understand the complexity of all the enzymes and regulatory components associated with the circadian clock of the plant.”
Cushman’s team has collected vast omic datasets from CAM plants. He estimates that several hundred genes coordinate the process, turning each other on and off and producing proteins that interact with each other and influence other genes.
His team is trying to engineer the plant Arabidopsis (a weed often used for genetic experiments) to use CAM by inserting the relevant genes into the plant. If they can get it to work in this small lab plant, the researchers want to do the same in poplar trees to help grow biofuel sources in harsh environments not usually suitable for agriculture. Someday, Cushman hopes, the technology will help food crops grow better in arid climates.
Better than a hunch
So far, collecting and integrating omic data is largely restricted to the lab — the work is still expensive and time-consuming. But scientists like Snyder, who diagnosed himself with type 2 diabetes, think that someday people could regularly collect these sorts of data to track changes in their health over time.
The Stanford geneticist began by analyzing his own genome. Then he started measuring his proteome, metabolome and other omic data at regular intervals to develop what he termed his “personal omics profile.”
Certain mutations in his genome suggested that he was at risk for developing diabetes from the get-go. He was surprised, but didn’t take action. Nearly a year into his experiment, though, changes in his other omic data suggested that his body was no longer using glucose properly. His blood sugar was elevated and so was a type of hemoglobin that increases with uncontrolled diabetes, according to a report in Cell by Snyder and three dozen colleagues in 2012. Those aren’t new measures — they’re exactly what a doctor would test in someone with diabetes symptoms.
But Snyder had no symptoms. He had just had a nasty cold, which he now thinks may have triggered the onset of diabetes because he was already genetically at risk.
Because he spotted the changes early and his doctor confirmed he had diabetes, Snyder was able to fend off symptoms by changing his diet and exercise habits — which was reflected in the follow-up data he collected.
He monitored how other biological measurements (molecules that standard medical diagnostic tests might not even look at) changed with the onset of his diabetes and the lifestyle changes he made in response. That information might be valuable for doctors hoping to detect the onset of diabetes in patients before symptoms appear. So now his lab is tracking omic data from 100 people, most of whom have elevated blood sugar levels, but have not yet been diagnosed with diabetes. Snyder wants to see whether viral infections trigger diabetes in other people, or whether his case is an isolated incident.
Snyder is still tracking fluctuations in his own data over time, too. He thinks it’s a powerful tool for personalized medicine because it shows in real time how an individual responds to disease and other stressors.
“You’ll be able to start linking disease to treatment to outcomes,” Snyder says. “It becomes what I call data-driven medicine, instead of hunch-driven medicine.”
Making sense of this sort of data isn’t easy. “No story is the same,” Snyder says. “We see a lot of strange stuff that we don’t know how to interpret yet.”
But collecting detailed omic-level data about individuals over time could still be useful, he says. By collecting more data from healthy people, doctors and scientists could get a better sense of what’s normal. And tracking fluctuations in individual patients’ data over time can show what’s typical for that particular person. Snyder thinks that might be more valuable than knowing what’s normal for people in general. Someone monitoring these biological signs might notice molecular changes — as he did — before they cause troublesome physical symptoms.
For the multi-omic future that Snyder and others envision, scientists will need a greater ability to store and wrangle massive amounts of data than they possess today. And they’ll need ways to test the networks they uncover. But the potential payoff is enormous: A response map that can display the intertwining routes between genes and outcomes, as well as how distant red lights, speed bumps and construction zones play off each other to shift those paths.